In the world of finance, Wall Street has long been synonymous with high-stakes trading, deep analysis, and complex decision-making. Yet, as the industry faces an ever-expanding universe of data and increasing pressure to optimize returns, a quiet revolution is taking place—one powered by artificial intelligence (AI). AI is gradually infiltrating every corner of financial services, from portfolio management to risk assessment and even the very algorithms that drive trading. This transformation is not only reshaping how financial firms operate but also redefining the relationship between human intuition and machine-driven decisions.
AI-driven tools are now an indispensable part of financial decision-making. For institutions that manage billions of dollars in assets, AI offers an unprecedented ability to process vast amounts of data, identify patterns, and make predictions with speed and accuracy that far exceed human capabilities. But as these intelligent systems take on a larger role, questions around ethics, transparency, and regulation are emerging. In this article, we’ll explore how AI is transforming Wall Street, examine the advantages it offers over traditional methods, and consider the challenges and opportunities that lie ahead for the future of financial technology.
Integration of AI in Portfolio Management and Risk Assessment
Portfolio management and risk assessment have always been critical elements of successful investing. Traditional methods often relied on expert analysis, historical data, and the subjective judgment of portfolio managers. But with AI, these processes have become far more dynamic, responsive, and data-driven. AI tools are now being employed to optimize asset allocations, manage risk, and predict market movements with remarkable precision.
AI in Portfolio Management
At the heart of AI’s integration in portfolio management lies its ability to make data-driven decisions. AI algorithms are designed to analyze vast amounts of financial data—ranging from market trends to economic indicators and corporate earnings reports—and generate actionable insights that human managers may overlook. By using machine learning (ML) techniques, these systems continuously evolve, adapting to new data as it becomes available. As a result, portfolio managers can rely on AI to assist in optimizing portfolio performance by recommending adjustments that align with specific investment goals, such as maximizing returns or minimizing risk.
One example of AI’s growing influence in this domain is the rise of robo-advisors, automated platforms that use algorithms to provide financial advice and manage investments. These tools, once considered niche, have gained significant traction, particularly in retail investment. Robo-advisors like Betterment and Wealthfront offer low-cost, personalized financial planning and asset management services that appeal to a broad audience. By leveraging AI, these platforms can continuously monitor and adjust portfolios, ensuring they remain aligned with the investor’s goals and risk tolerance.
However, AI’s potential goes beyond just automating existing processes. Advanced machine learning algorithms can now analyze patterns in market data that were previously undetectable, allowing for more accurate predictions of market behavior. Hedge funds and institutional investors are increasingly relying on these systems to make real-time trading decisions, adjusting their portfolios dynamically as market conditions evolve.
AI in Risk Assessment
Risk management is an area where AI has proven to be particularly valuable. In traditional risk models, the inputs were often limited to historical price data and simplistic forecasts. AI, on the other hand, allows for a more nuanced understanding of risk by incorporating a broader range of variables, such as geopolitical events, weather patterns, or even social media sentiment.
Using natural language processing (NLP), AI tools can analyze news articles, earnings calls, and social media posts to gauge public sentiment about a particular stock or sector. This real-time data processing enables risk managers to identify emerging risks much earlier than traditional methods would allow. Moreover, machine learning models can identify correlations between seemingly unrelated factors, uncovering potential risks that might not be visible through conventional analysis.
For example, BlackRock, one of the largest asset management firms in the world, has developed an AI-driven risk management system known as Aladdin. Aladdin integrates data on portfolio risk, market volatility, and economic indicators, allowing investors to make more informed decisions about asset allocation and risk mitigation. This system is widely used by institutional investors, including pension funds, insurance companies, and sovereign wealth funds, helping them navigate the complexities of global markets with more precision.
Advantages Over Traditional Analytical Methods
AI’s rise in finance is largely due to its ability to outperform traditional analytical methods in several key areas. These advantages—speed, accuracy, scalability, and adaptability—are quickly making AI an essential tool for any institution that deals with large volumes of data or requires precision in its decision-making processes.
Speed and Efficiency
One of the biggest advantages of AI is its ability to process data at speeds far beyond human capability. In financial markets, where time is of the essence, the ability to analyze and respond to real-time data quickly can provide a significant competitive advantage. Machine learning models can sift through vast amounts of financial data in milliseconds, spotting trends and making predictions that would take human analysts hours or even days to uncover.
For example, algorithmic trading relies on AI to automatically execute trades based on pre-programmed strategies and real-time data inputs. These algorithms can place orders and adjust positions far faster than human traders, enabling firms to capitalize on short-lived market opportunities. According to Research and Markets, the global market for algorithmic trading is expected to grow at a CAGR of 11.23% from 2021 to 2026, reflecting the increasing reliance on AI-driven systems in trading.
Accuracy and Precision
AI algorithms are also far more accurate than traditional methods, especially when it comes to detecting patterns in large datasets. Traditional financial analysis often relies on human judgment, which can be subjective and prone to error. AI, by contrast, is data-driven and objective, making decisions based on statistical models and historical data rather than personal biases or heuristics.
For example, machine learning models can predict stock price movements with much greater precision than traditional methods. By analyzing historical price data, trading volumes, and macroeconomic indicators, these algorithms can identify patterns that are too complex for human analysts to detect. This increased accuracy allows investors to make better-informed decisions, optimizing returns while reducing risk.
Scalability and Adaptability
AI’s ability to scale is another key advantage over traditional methods. As data volume grows, AI systems can handle increasingly complex datasets without a loss of performance. This is particularly important in financial services, where the sheer amount of data—from market prices to geopolitical events—can overwhelm traditional analytical tools.
Furthermore, AI systems are highly adaptable. As they are exposed to more data, they continuously learn and improve, allowing them to adjust to changing market conditions. This adaptability is particularly valuable in volatile markets, where predicting future trends with traditional models can be challenging.
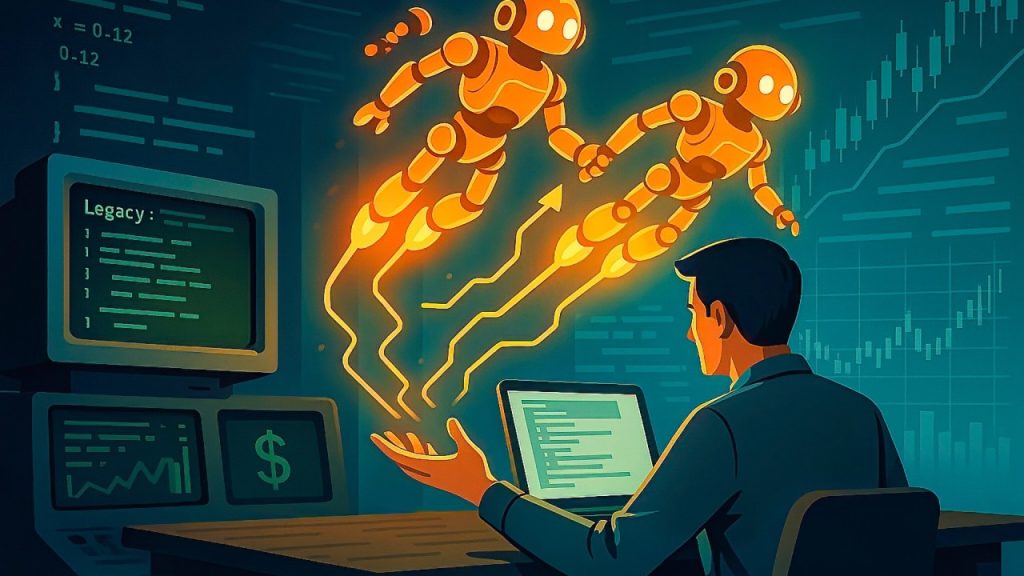
Ethical Considerations and Regulatory Challenges
While AI offers significant advantages, it also raises important ethical and regulatory concerns that must be addressed. As financial institutions increasingly rely on AI to make decisions that impact the lives of millions of people, issues of transparency, accountability, and fairness come to the forefront.
Transparency and Accountability
One of the biggest ethical concerns with AI in finance is the “black-box” nature of many machine learning models. While these models can make highly accurate predictions, their decision-making processes are often opaque, making it difficult for regulators and investors to understand how decisions are being made. This lack of transparency can lead to questions of accountability, especially in cases where AI-driven decisions result in financial losses or market disruptions.
To address this issue, regulators are pushing for greater transparency in AI systems. The European Union’s Artificial Intelligence Act and the U.S. Federal Reserve’s recent proposals on AI and fintech both emphasize the importance of making AI systems more interpretable and explainable. Financial institutions will need to ensure that their AI systems are not only accurate but also understandable to regulators and customers alike.
Bias and Fairness
Another concern is the potential for bias in AI models. If an AI system is trained on historical data that reflects existing biases—such as gender, racial, or socio-economic biases—the model may perpetuate these biases in its decision-making. In the context of finance, this could lead to discriminatory lending practices, unequal access to financial services, or biased investment decisions.
To mitigate this risk, financial institutions must ensure that their AI models are trained on diverse, representative datasets and subject to regular audits for fairness. By doing so, they can help ensure that AI-driven decisions are fair and equitable for all stakeholders.
Regulatory Oversight
As AI continues to play a larger role in the financial industry, regulatory oversight will be crucial in ensuring that these technologies are used responsibly. While AI holds the potential to enhance efficiency and profitability, it also poses risks that could disrupt financial markets or harm consumers. Regulators must strike a balance between fostering innovation and protecting investors and consumers from the potential downsides of AI-driven financial systems.
Future Prospects for AI-Driven Financial Tools
The future of AI in financial services looks bright, with the technology poised to continue transforming how markets operate. In the coming years, we can expect even more sophisticated AI systems that can make more precise predictions, optimize portfolios in real-time, and provide personalized financial advice to investors.
AI will also likely play a significant role in financial inclusion, offering more people access to affordable financial services. As AI becomes more advanced, it will be able to tailor financial products to individuals’ needs, regardless of their credit history or financial background. This could be particularly beneficial for underbanked or underserved populations around the world.
Ultimately, AI has the potential to unlock unprecedented opportunities for both investors and financial institutions. However, it will also require careful regulation and ethical oversight to ensure that its benefits are realized in a responsible, transparent, and equitable manner.
Conclusion
AI is quietly reshaping Wall Street, introducing new ways to manage portfolios, assess risks, and make financial decisions. As these technologies continue to evolve, their impact
on the financial industry will only grow more profound. While AI offers tremendous advantages in terms of speed, accuracy, and adaptability, it also presents challenges related to transparency, bias, and regulatory oversight. By addressing these concerns, the financial industry can harness the power of AI to drive innovation, enhance financial services, and create a more inclusive financial system for the future.